Data Science Framework: Our navigation system for your successful data-based optimisation!
Would you adapt your manufacturing process if a chatbot made suggestions for improvement? Now that ChatGPT is becoming more and more part of our everyday lives, the pharmaceutical industry will also have to deal with artificial intelligence (AI). In this article, we will show you how our data science framework creates a structured basis for your data science projects in order to be prepared for a possible future with AI.
What do buzzwords such as "data mining" or "machine learning" trigger?
The world has changed. What was once a dream of the future will be reality in 2023. Neural networks, whose algorithms have been created in such a way that they can learn from situations, offer us unimagined possibilities. We are probably all already using these new technologies successfully: we ask our smart watch for the weather, firefighters practise dangerous situations with the help of virtual reality and, thanks to autonomous vehicles, we are now just passengers in our cars.
We assume that the topic of artificial intelligence will also play an increasingly important role in our industry. However, first, proven foundations must be created through data science in order to take the next step towards AI.
Will we no longer need the human factor in the future?
Of course, humans still play an important role in artificial intelligence. This is also the case in our CTE Data Science Framework. Let's break down buzzwords such as "data mining", "machine learning", "neural networks" or even "artificial intelligence" and look at answers that will explain "how". How can we get to artificial intelligence while taking into account the regulatory requirements that apply in the pharmaceutical industry? It's not that simple.
As experts in process automation, we have been driven by the 'how' for over 30 years. Automated solutions shape our everyday lives. This drive has led us to develop a standardised process that allows us to make data-driven decisions with the help of data science. We are convinced that pure data storage does not exhaust the potential. Data should be interpreted if we want to unearth the treasures at the bottom of the sea. Let's dive in together and find out where data science can create added value on the road to artificial intelligence!
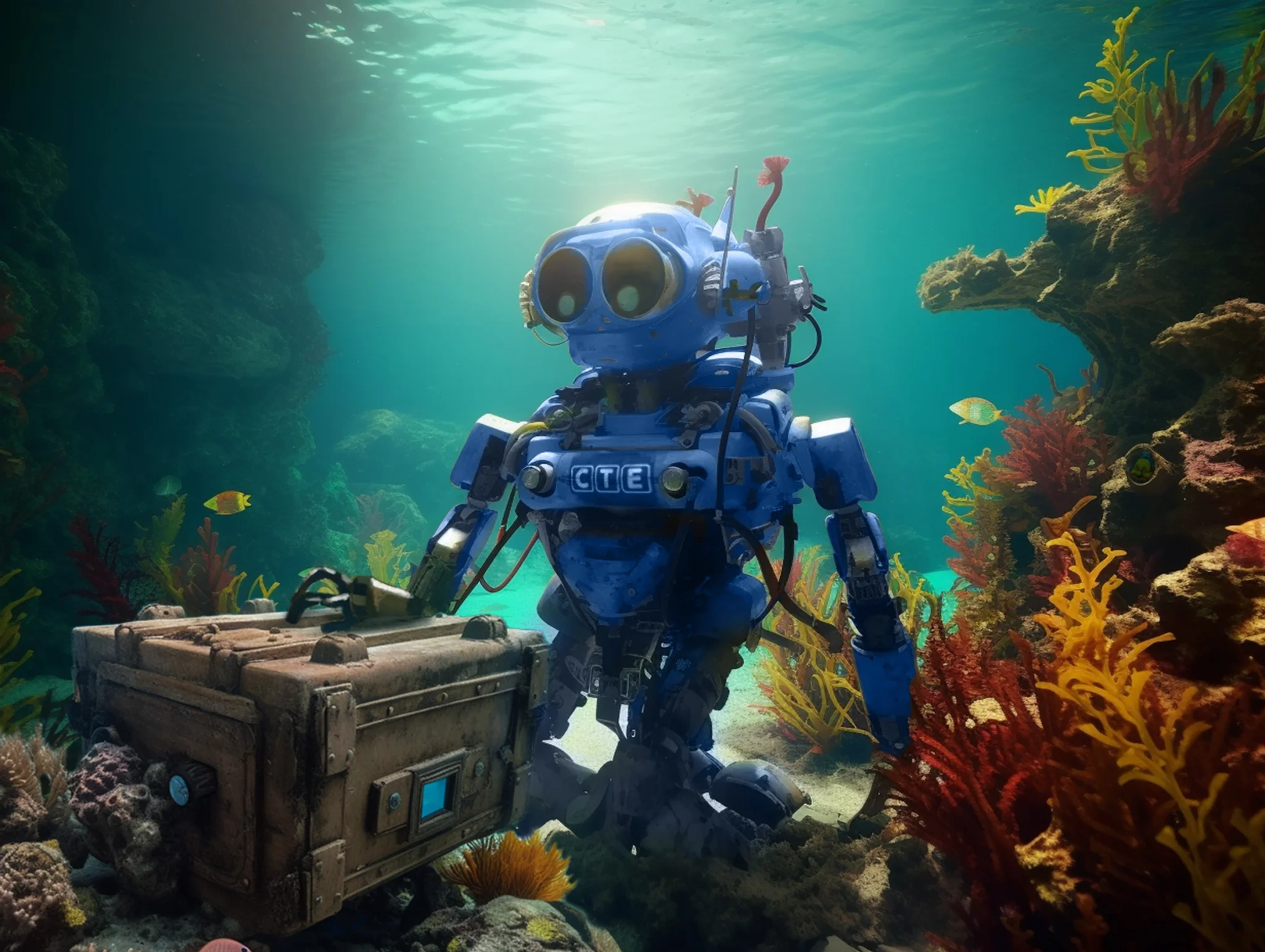
Where can data science create real added value?
It's not about rationalising jobs due to artificial intelligence. We believe that people will continue to play an important role, but will be able to concentrate on what's really important. People are still required in all areas of engineering, for example to develop basic principles or make decisions.
Data science can create added value in other areas: In automating and improving the quality of reports and evidence in the GMP environment. Or in the identification and storage of process know-how. In the even of a shortage of skilled workers or if an expert leaves the company, knowledge is lost. This doesn't happen with AI: once the neural network has learned, the knowledge it has acquired is never forgotten.
With our data science framework, we create the basis for data-based optimisation of the entire manufacturing and operating processes. Before data-based optimisation can be implemented, data must be collected, stored, contextualised and correlated.
As experts in the industrial automation of production systems, we focus on recognising added value. Be it in the manufacturing process or in ancillary processes or activities. The aim is to unleash unimagined potential and save valuable time. Your profitability and process reliability are our core tasks.
Recent figures from a study by Dimension Research Study show the three most common problems that companies face in connection with data: "Errors in data" (66%) "Not enough data" (51%) and "Data not available in a suitable form" (50%).
Moreover, McKinsey has determined that 45% of large IT projects run out of budget. To counter this tendency, our approach is to avoid tackling everything at once, but instead, to implement the set goals iteratively. In this way, initial goals and perhaps, valuable findings, are achieved earlier, the effort is more manageable and costs may even be reduced thranks to the initial findings.
Our CTE Data Science Frameworks are designed to support you in your IT project and relieve you of work so that you can concentrate on your content and project goals.
Admittedly, this is not necessarily rocket science. But on the path from big data to data science to artificial intelligence, these steps are essential. The pharmaceutical industry has a huge advantage: it has already been collecting data for many years, as this is required by the authorities. Now this data is lying as a treasure at the bottom of the sea and should be recovered as such. Imagine what new insights you could gain from your data?
Once we have defined the framework for your project together, you will be provided with a dashboard on which you can easily monitor the connections. Until now, your data may have been in the form of Excel spreadsheets, text files or even printouts, which is probably rather confusing. The beauty of our CTE Data Science Framework is that it delivers a result after each iteration, allowing you to decide after which run the end has been reached. However, you can also resume work at any time in order to build on the existing result.
Especially in the GMP environment - i.e. in a highly regulated environment - the hurdle of working with data to be collected seems to be higher than perhaps in other industries... For this reason, AI is often not even given a chance. Wrongly so! Our goal is to increase the usefulness of this data and perhaps one day give AI algorithms a chance to uncover unexpected insights.
Let's start a pilot project, and let's become pioneers in the GMP environment!
We have arguments, we have the CTE Data Science Framework, we just need real data from the GMP environment. We want to transfer our theory into practice. However, finding (GMP) customers who are willing to start a pilot project with us is not that easy. Too expensive, too complex, too little outcome, too many application errors were just some of the arguments against our pilot project. But we are convinced that "Data Science" with our CTE Data Science Framework paves the way to artificial intelligence.
The aim of a pilot project with you is to implement our data science framework, from the formulation of objectives to the application and optimisation of data. In addition, we want to determine how data-based optimisation can be further automated in a regulated environment.